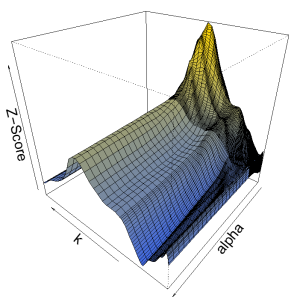
Robust computational methods for proteome data analysis
Proteome profiling provides a compelling option for biomedical studies and biomarker discovery thanks to its capabilities beyond genomic studies. To enable translating the high-throughput proteome data to biomedical knowledge, we develop robust computational methods and tools for proteome data analysis, with focus on reproducible analysis of mass spectrometry proteomics and longitudinal profiling data. By combining multidisciplinary expertise in statistical and machine learning, proteomics and clinical research, the aim is to accelerate development of improved diagnosis, prognosis, and treatment strategies for complex diseases, such as diabetes.
Selected publications from the lab: Suomi et al. (2022) Immunol Lett, Hong et al. (2022) Brief Bioinform, Välikangas et al. (2018) Brief Bioinform, Saraei et al. (2018) Bioinformatics, Suni et al. (2018) Bioinformatics, Suomi et al. (2017) PLoS Comput Biol
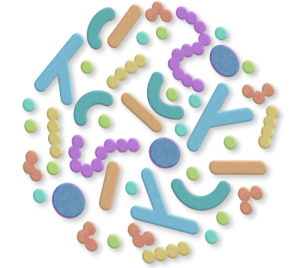
Next-level analysis of complex microbial samples using metaproteomics
Metaproteomics is an emerging research area to analyse complex microbial communities at protein level. The major benefit over the widely used metagenomics approaches is the ability to directly reveal the functionality of the microbiota. We develop tools for next-level metaproteome analysis of complex microbial samples, with focus on data-independent acquisition mass spectrometry, which we were recently the first to show can be successfully applied to analyse complex metaproteome samples. Our biomedical focus is on the characterization of the human gut microbiome, which has been associated with various health and disease states.
Selected publications from the lab: Pietilä et al. (2022) ISME Commun, Aakko et al. (2020) J Proteome Res
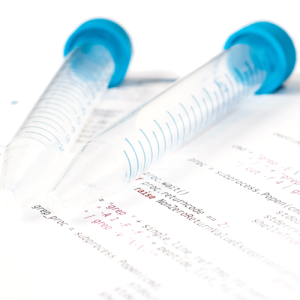
Robust computational methods for epigenome and multi-omics data analysis
Epigenomics refers to the study of gene regulation not attributable to alterations in the DNA sequence, with the main epigenetic mechanisms including DNA methylation, histone modifications, and regulation by non-coding RNAs. High-throughput epigenome profiling together with other multi-omics data has shown high potential to reveal novel candidate biomarkers and disease mechanisms. To make the most out of these data, we develop robust computational tools for their interpretation in multidisciplinary biomedical research collaboration, with focus on reproducible identification of changes in DNA methylation and chromatin modification states, as well as robust discovery of novel long non-coding RNAs and their regulatory mechanisms.
Selected publications from the lab: Ammunét et al. (2022) Brief Funct Genom, Rytkönen KT et al. (2022) iScience, Faux et al. (2021) NAR Genom Bioinform, Suni et al. (2020) Epigenomics, Khan et al. (2020) J Clin Invest
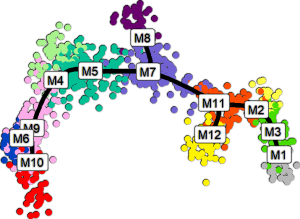
Computational tools for single-cell data analysis and integration
Versatile tools are needed to help interpret the vast and increasingly complex data generated by single-cell technologies, which have recently attracted wide interest thanks to their capabilities beyond the more conventional studies of bulk cell populations. We aim to optimize the single-cell data analysis and to develop new statistical and machine learning methods for the emerging new technologies and complex design settings, including longitudinal, spatial, and multi-omics single-cell data. The aim is to enable prediction of molecular signatures and regulatory networks in various healthy and disease states in a reproducible manner.
Selected publications from the lab: Kleino et al. (2022) Comput Struct Biotechnol J, Junttila et al. (2022) Brief Bioinform, Smolander et al. (2021) Bioinformatics, Smolander et al. (2021) Bioinformatics, Adossa et al. (2021)Comput Struct Biotechnol J, Jaakkola et al. (2017) Brief Bioinform
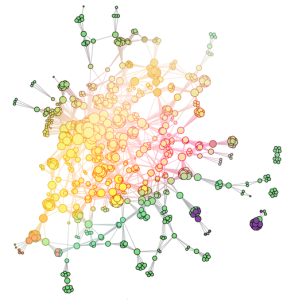
Computational systems immunology applications: human T cells and immune-mediated diseases
A key question in today’s systems immunology is the ability to transform high-resolution multi-omics data into useful knowledge about our immune system and its disorders, with both the size and complexity of the data constantly increasing. Our goal is to apply the novel computational methods that we develop to enable new discoveries in human T cell regulation and new understanding about the development of human immune-mediated diseases, such as the autoimmune type 1 diabetes, in close collaboration with biomedical and clinical research teams.
Selected publications from the lab: Suomi et al. (2022) Diabetes Care, Välikangas et al. (2022) Front Endocrinol, Shetty et al. (2022) Nucleic Acids Res, Khan et al. (2022) Front Immunol,Tripathi et al. (2019) iScience, Lietzen et al. (2018) Diabetologia
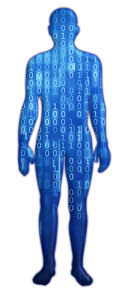
Artificial intelligence in the analysis of digital health data: early prediction of disease and treatment risks
Artificial intelligence together with the growing amounts and diversity of digital health data provide excellent opportunities for early prediction of disease and treatment risks, including both molecular omics profiling data as well as data from various other sources, such as imaging, electronic medical records, or wearable devices. By combining multidisciplinary expertise, we aim to enable robust, clinically feasible prediction of individualized disease or treatment risks dynamically before the clinical manifestation of the disease or before starting the treatment. The aim is to develop models that are both accurate as well as interpretable and easy to use in practice to accelerate the development of improved diagnosis, prognosis and treatment strategies for complex diseases, such as diabetes and cancer.
Selected publications from the lab: Venäläinen et al. (2022) Cancer Med, Sieberts et al. (2021) NPJ Digit Med, Mahmoudian et al. (2021) Bioinformatics, Venäläinen et al. (2020) J Hypertens, Fourati et al. (2018) Nat Commun, Seyednasrollah et al. (2017) Eur Urol
Our research is possible thanks to our supporters
Our projects have received funding from the European Research Council (ERC), the European Union’s Horizon 2020 research and innovation programme, the Juvenile Diabetes Research Foundation (JDRF), Academy of Finland, Business Finland, and the Sigrid Juselius Foundation.
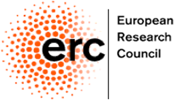
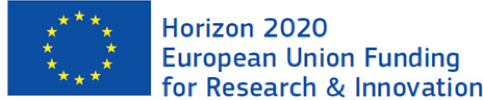
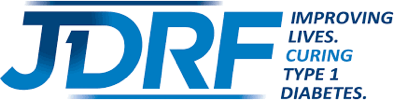
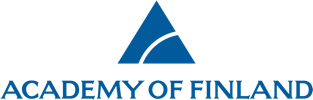
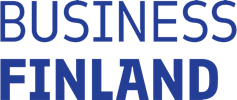
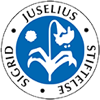